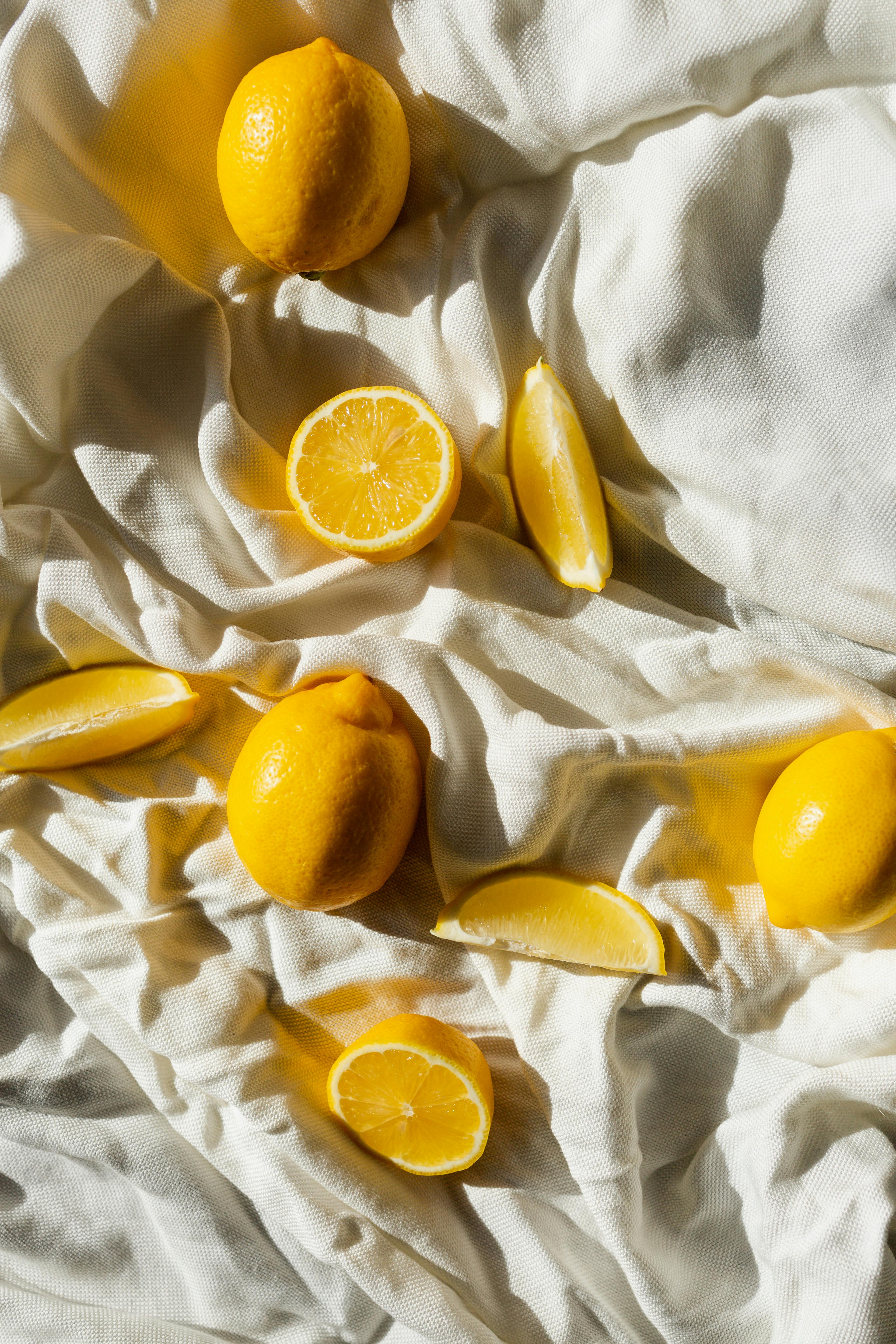
How to Effectively Calculate Error for Accurate Data Analysis in 2025
Understanding Error in Data Analysis
Calculating **error** is a crucial step in the realm of data analysis, especially as we venture into the new challenges of 2025. In the context of data science, **error** refers to the difference between the actual value and the estimated value produced by a model. Understanding various types of **error**—such as systematic and random errors—provides a solid foundation for developing effective strategies for precise analysis. Moreover, recognizing how to interpret these **errors** can lead to informed decisions, better models, and ultimately improved outcomes. It's essential for analysts to familiarize themselves with the implication of **errors** and adopt methods to minimize them through meticulous calculations.
Types of Error in Data Analysis
There are primarily two types of **error** that analysts must contend with: systematic and random errors. Systematic **errors** occur consistently in the same direction and can skew results, often due to flawed measurement processes or equipment. For example, if a thermometer is inaccurately calibrated, all temperature readings will display a consistent **error**. On the other hand, random **errors** are unpredictable and vary in magnitude and direction, causing fluctuations that complicate precise analysis. Understanding these distinctions aids in troubleshooting and refining data collection techniques to enhance the integrity of results in your analytical endeavors.
Common Formulas for Error Calculation
To effectively calculate **error**, it's vital to use established formulas that can gauge accuracy quantitatively. One of the most widely used is the Mean Absolute Error (MAE), which sums up the absolute values of the **errors** between predicted and observed values, dividing by the number of observations. The formula is expressed as: MAE = (1/n) * Σ |Actual - Predicted|. Another important measure is the Root Mean Square Error (RMSE), which squares the individual **errors**, averages them, and takes the square root. The formula for RMSE is RMSE = √((1/n) * Σ (Actual - Predicted)²). These frameworks provide quick insights into the model's predictive accuracy, critical for decision-making.
Impact of Error on Data Validity
The impact of **error** on data validity can be profound, influencing the reliability of outcomes derived from analysis. High **error** margins can lead to misinterpretations, causing stakeholders to make decisions based on flawed insights. To mitigate this impact, analysts should regularly review the sources of **errors** and seek methods to enhance data collection processes. Regular recalibration of measuring tools, while training personnel in accurate techniques, can significantly reduce the potential for systematic **errors**. Furthermore, it is essential to perform multiple analyses and average the outcomes, enabling analysts to fine-tune the overall results.
Case Study: Reducing Error in Marketing Data Analysis
A company once faced challenges computing return on investment due to high **error** rates in their marketing analytics. They began implementing systematic checks for **errors**, adopting tools that automatically calibrated data inputs and monitored discrepancies in real-time. By understanding the sources of their systematic **errors**, like outdated campaign performance metrics, the company managed to adjust their methodologies, which resulted in a significant reduction in ambiguity around their **error** margins. This example illustrates how proactive measures in calculating and understanding **errors** yield metrics that directly contribute to the company’s strategic decisions and profitability.
Best Practices for Error Calculation
To enhance the accuracy and effectiveness of **error** calculations, analysts should adhere to several best practices: consistently validate data sources, standardize equipment settings, apply statistical methods for evaluating **errors**, and leverage advanced software tools for analysis. Additionally, establishing a routine for frequent audits of data sets ensures that any **error** sources can be quickly identified and rectified. A combination of diligence and the right analytical techniques sets the foundation for achieving high levels of data integrity in 2025.
Future Trends in Error Calculation
As the field of data analysis continues to evolve, trends in **error** measurement will also advance significantly. In 2025, we anticipate the integration of artificial intelligence and machine learning techniques in error analysis, providing more precise adjustments in real-time. Automation tools will reduce human handling **errors**, streamlining processes for more accurate results. Furthermore, there may be increased emphasis on data ethics, with standards asserting the need for transparency about conceivable **errors** in data reporting. These forecasts speak to the importance of adapting to new methodologies, which will ultimately improve data analysis practices.
Technology's Role in Mitigating Errors
The role of technology in potentially mitigating data **errors** cannot be overstated. With the advancement of computational tools, analysts will keep shifting towards intelligent algorithms that predict and check for **errors** based on historical data patterns. Rapid error detection systems can now alert analysts in real-time to significant discrepancies, allowing immediate adjustments. Additionally, leveraging cloud computing enables larger datasets to be processed accurately, reducing human involvement with repetitive tasks that might introduce **errors**. Employing robust technological solutions holds the promise of vastly improving the accuracy of calculations.
Predictive Errors: Analyzing Future Possibilities
Looking ahead, predictive **error** analysis will become a standard element in strategic planning across industries. Forecasting models that integrate recent trends will help businesses anticipate potential discrepancies, ensuring they are better prepared for future uncertainties. As organizations gather more data, understanding these predictive **errors** will aid in crafting models that evolve continuously. By applying advanced machine learning techniques, businesses can analyze patterns in historical **errors**, understanding them as key indicators of future challenges, thus building resilience in their analytical processes.
Key Takeaways
- Understanding different types of error - systematic and random - is essential for accurate data analysis.
- Utilizing robust formulas like Mean Absolute Error (MAE) and Root Mean Square Error (RMSE) will enhance precision.
- Regularly reviewing data sources and analysis methods can lead to improved data validity.
- Adopting technology will play a crucial role in automating error detection and enhancing accuracy.
- Predictive errors can inform strategic planning across domains, making businesses more resilient.
FAQ
1. What is the difference between systematic and random errors?
Systematic **errors** are consistent and predictable discrepancies often caused by inherent problems within the measurement system, affecting results in the same direction. Conversely, random **errors** vary in magnitude and direction due to unpredictable factors, complicating the analysis of results. Understanding these differences is key to mitigating their effects on data outcomes.
2. How can I reduce error in my data collection method?
To reduce **error** in your data collection, standardize procedures and regularly calibrate your measuring tools. Train personnel on proper techniques and use statistical tools that highlight discrepancies. Performing audits of your data collection process will also identify sources of **error**, allowing for timely improvements to methods.
3. What are the most common formulas for calculating error?
Some of the most common formulas to calculate **error** include Mean Absolute Error (MAE), Mean Squared Error (MSE), and Root Mean Square Error (RMSE). Each comes with its strengths and weaknesses, but applying them will help quantify the accuracy of data against the actual values, informing better decision-making.
4. How can technology enhance error analysis?
Technology enhances **error** analysis by providing tools for automation, making real-time detection of discrepancies possible. Advanced algorithms analyze vast datasets with minimal human interference, increasing accuracy. This not only saves time but considerably reduces human **error** introduced through manual processes.
5. What are predictive errors and why are they significant?
Predictive **errors** are discrepancies forecasted based on historical data patterns. They are significant because they help organizations prepare for potential challenges in future analysis. By understanding and incorporating predictive **error** analysis, businesses can adapt their strategies effectively and enhance resilience.